Agent Q represents a significant advancement in AI technology. It combines Monte Carlo tree search and direct preference optimization to excel at complex, multi-step tasks. This innovative approach allows AI to navigate real-world scenarios with unprecedented success, outperforming traditional AI models and human benchmarks in tasks like online reservations.
- 1. Introduction to Agent Q
- 2. The Challenge of Complex Decision-Making in AI
- 3. How Agent Q Works: MCTS and DPO
- 4. Testing Agent Q: From Simulation to Real-World Applications
- 5. Performance Comparison: Agent Q vs. Traditional AI
- 6. The Self-Improving Nature of Agent Q
- 7. Challenges and Future Developments
- 8. Implications for AI in Everyday Life
- For More
1. Introduction to Agent Q
In the rapidly evolving world of artificial intelligence, a new player has emerged that promises to revolutionize how AI tackles complex, multi-step tasks. Meet Agent Q, an innovative AI system developed by the AGI company in collaboration with Stanford University. This groundbreaking technology represents a significant leap forward in AI’s ability to make decisions in unpredictable, real-world environments.
Agent Q isn’t just another language model; it’s a sophisticated decision-making system designed to navigate the complexities of tasks that have traditionally stumped even the most advanced AI. From planning international trips to making restaurant reservations, Agent Q showcases unprecedented adaptability and success in scenarios requiring careful consideration and sequential decision-making.
2. The Challenge of Complex Decision-Making in AI
While impressive in their ability to process language and perform specific tasks, traditional AI models often fall short when faced with complex, multi-step challenges. These models typically rely on static datasets and struggle to adapt to dynamic, unpredictable environments. For instance, booking a flight or navigating an e-commerce website can prove challenging for conventional AI due to constantly changing variables and the need for real-time decision-making.
This limitation has long been a stumbling block in developing versatile AI systems. Making decisions over several steps, especially in unpredictable environments like the web, requires a level of adaptability and foresight that goes beyond simple pattern recognition or language processing.
3. How Agent Q Works: MCTS and DPO
At the heart of Agent Q’s capabilities lies a powerful combination of two advanced techniques: Monte Carlo Tree Search (MCTS) and Direct Preference Optimization (DPO). This fusion allows Agent Q to explore different possible actions and learn from its experiences in a way that mimics human problem-solving.
MCTS, a previously successful method in game-playing AI, helps Agent Q explore different possible actions and determine which will likely lead to the best outcome. This approach allows the AI to think several steps ahead, considering various scenarios before deciding.
Complementing MCTS is DPO, which enables Agent Q to learn from its successes and failures. Unlike traditional reinforcement learning, which relies on clear win/lose outcomes, DPO allows for a more nuanced understanding of the decision-making process. It analyzes the entire journey, identifying which decisions were beneficial and which weren’t, even if the result was successful.
4. Testing Agent Q: From Simulation to Real-World Applications
The researchers behind Agent Q put their creation through its paces, starting with a simulated environment called WebShop. This controlled setting mimicked the complexities of real e-commerce sites, providing a challenging yet safe space for Agent Q to demonstrate its capabilities. The results were impressive, with Agent Q significantly outperforming other AI models in completing tasks like finding specific products.
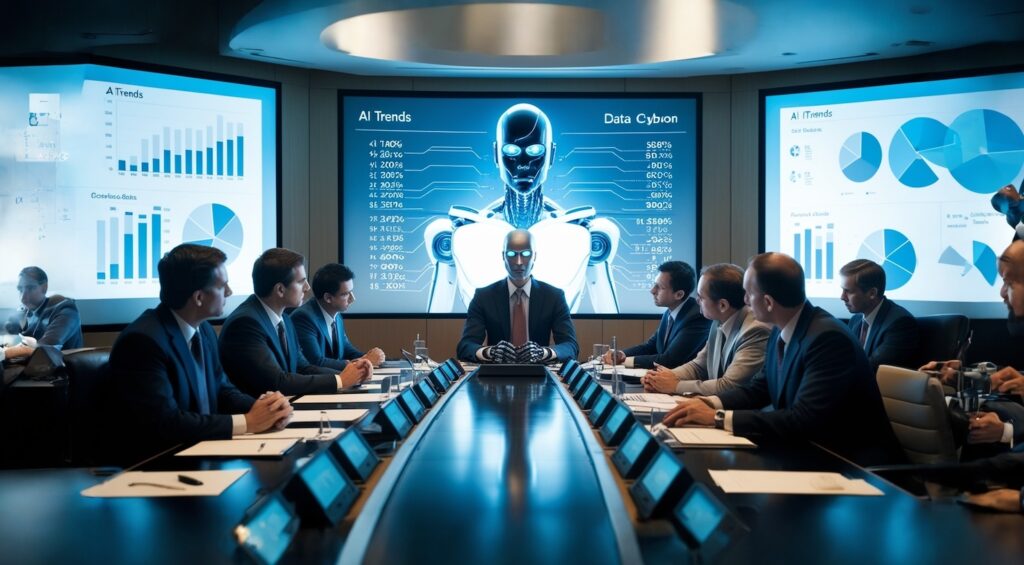
However, the test came when Agent Q was unleashed on real-world tasks. The researchers challenged it to book a table on OpenTable, a popular restaurant reservation website. While seemingly simple, this task involves navigating through varying options depending on time, location, and restaurant availability – a complex endeavor that even humans sometimes find frustrating.
5. Performance Comparison: Agent Q vs. Traditional AI
The performance of Agent Q in real-world applications was nothing short of remarkable. When tasked with making reservations on OpenTable, the best previous AI model (LLaMA 3 70B) had a success rate of just 18.6%. In stark contrast, after just one day of training, Agent Q achieved a success rate of 81.7%.
Even more impressively, when equipped with the ability to perform online searches to gather additional information, Agent Q’s success rate soared to an astonishing 95.4%. This level of performance not only surpassed other AI models and exceeded average human performance, which hovers around 50% for the same task.
6. The Self-Improving Nature of Agent Q
One of the most fascinating aspects of Agent Q is its ability to learn and improve continually. Unlike traditional AI models that remain static after training, Agent Q utilizes a self-critique mechanism. After each action, it pauses to evaluate its performance, guided by an AI-based feedback model that ranks possible actions and suggests improvements.
This self-reflection allows Agent Q to fine-tune its decision-making in real-time, making it more reliable and effective with each task it completes. The system also employs a replay buffer, enabling it to learn from past actions without repeating the same mistakes, enhancing its efficiency and accuracy over time.
7. Challenges and Future Developments
Despite its impressive performance, the development of Agent Q is not without challenges. The researchers acknowledge the potential risks of allowing an AI to operate autonomously in sensitive environments. They are actively working on mitigating these risks, possibly through increased human oversight or additional safety checks.
The team is also exploring alternative search algorithms that could further enhance Agent Q’s performance. While MCTS has proven highly effective, other approaches may push the boundaries of AI reasoning and decision-making capabilities even further.
8. Implications for AI in Everyday Life
The implications of Agent Q’s capabilities are far-reaching. As AI systems become increasingly adept at handling complex, multi-step tasks with minimal supervision, we may see a shift in how we interact with technology in our daily lives. The potential applications are vast, from managing travel arrangements to navigating complicated online systems or tackling advanced tasks like legal document analysis.
Agent Q represents a significant step towards AI systems that can genuinely assist and augment human capabilities in meaningful ways. As these systems continue to evolve and improve, we may rely on them more frequently for tasks that require significant manual effort, potentially freeing up human resources for more creative and strategic endeavors.
In conclusion, Agent Q is a testament to the rapid advancements in AI technology. Combining innovative techniques like MCTS and DPO has achieved real-world problem-solving capability previously thought to be the exclusive domain of human intelligence. As we look to the future, it’s clear that AI systems like Agent Q will play an increasingly important role in shaping how we interact with technology and solve complex problems in our daily lives.
For More
Watch the AI Revolution 9-minute video “The AGI Company Presents Agent Q The AI Master of the Impossible.”